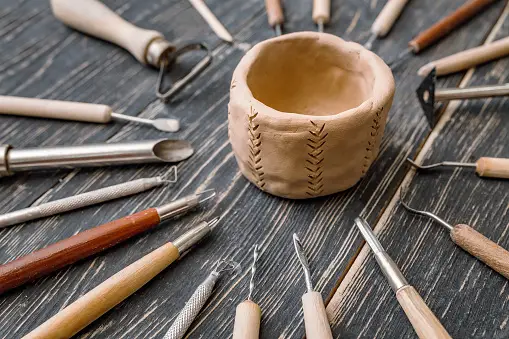
Dimensional Modelling in Data Warehousing
Dimensional Modelling (DM) is a method of designing databases optimised for faster data retrieval from a Data Warehouse, providing a powerful tool for users to analyse complex data and gain valuable business insights. The concept was first introduced by Ralph Kimball, in his book The Data Warehouse Toolkit. DM not only enhances data retrieval but also readability, organising data into a structure that is easy to understand and, unlike relational models, which focus on real-time data processing and reducing redundancy within the data, dimensional models are tailored for the efficient retrieval of numeric information, making them a great choice for Data Warehouse systems.
This article aims to describe the concepts, methods, benefits, and challenges of using dimensional modelling.
Core Concepts of Dimensional Modelling
Dimensional modelling is designed to simplify databases for general business intelligence users. At its core, the model uses two types of tables.
Fact tables: These tables contain quantitative data, such as metrics of business operations, that are numeric and measurable (e.g. sales revenue). The fact table typically contains keys that relate to dimension tables.
Dimension tables: These tables provide descriptive attributes for the data in fact tables, providing context for the quantitative data in fact tables. For instance, a dimension table could contain geographic location, time periods, products, or any other category by which the business is measured or categorised.
Why Dimensional Modelling?
Dimensional Modelling has many benefits that make it an effective method for designing a data warehouse. For example:
Simplified data retrieval - By organising data into streamlined dimension tables and fact tables, data retrieval and analysis are simplified, ultimately improving the usability and accessibility of your data. Dimensional models are designed with the user in mind, fitting seamlessly into pre-existing business processes.
Analytics - A dimensional model is useful for multidimensional data analysis, as the structure facilitates data exploration, allowing deeper insights into patterns and trends.
Quick querying - The denormalised structure of a dimensional model leads to improved query performance and overall increased efficiency for any reporting needs.
Adaptability - Dimensional modelling is flexible and adaptable and can accommodate any evolving business requirements, ensuring scalability as your organisation grows.
Designing a Dimensional Model
When setting out to design a dimensional model for a data warehouse, it’s important to consider some crucial elements to make sure the model fits your business goals before diving in too deep.
Identify business requirements - It is crucial to determine the specific business processes that the data warehouse would cover (e.g. Marketing, Sales, HR) based on the organisation’s data analysis requirements and data quality.
Identify the granularity - The granularity refers to the level of detail at which data is stored and is fundamental for data analytics and insights derived from the data. For instance, for long-term trend analysis, data stored monthly or quarterly may be preferable so it’s important to consider questions such as:
- How frequently should the data be stored and how often do you analyse trends?
- How would different departments use the data, what requirements do they have?
- Are there any specific business metrics that require more detailed data? What is the granularity of existing data processes?
Choosing relevant metrics for the fact table - A fact table should include keys to dimension tables and quantitative metrics that align with business objectives.
Incorporate attributes into dimension tables - Carefully choose relevant attributes to incorporate into the dimension table. For example product, store location, date, or time.
Choosing a schema - A schema refers to the logical structure between the tables in a database. Choosing the right schema is important for its impact on the efficiency and scalability of a data warehouse. It's best to keep in mind the business’s data management and analytics goals when choosing.
The Star Schema and Snowflake Schema are two common dimensional modelling structures you might come across. In a star schema, a fact table is surrounded by dimension tables in a manner that resembles a star. The design is simple, denormalised, and efficient for querying. On the other hand, a snowflake schema is a more normalised version of a star schema, where dimension tables are further normalised into sub-dimension tables. The result is more tables with fewer attributes, which helps to reduce data redundancy and improve storage efficiency.
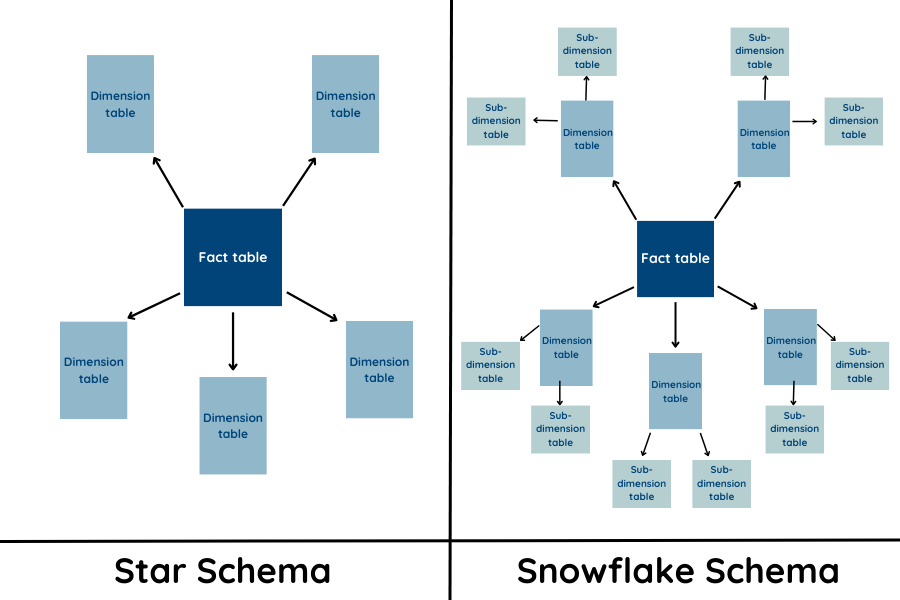
Challenges and Solutions in Dimensional Modelling
When delving into dimensional modelling, you may encounter some common challenges. The issue of large dimension tables can become a stumbling block regarding efficient data management and retrieval. Utilising sub-dimension tables can manage complexity while also optimising performance. This allows querying and data retrieval to become more streamlined and effective.
Balancing performance and complexity is another common challenge that warrants a closer look. It’s crucial to determine the best way to normalise data while keeping the dimensional model scalable in relation to operational processes. Striking the right balance is essential to ensure the design remains efficient yet manageable and lays the foundations for a robust and flexible model.
Finally, an issue to be mindful of is the matter of data changes. An attribute may change over time, and it is important to keep track of these changes. There are several approaches to this. For instance, overwriting the previous data, creating a new row and a new attribute to indicate the current active record or adding a new attribute column to keep track of the updated value of the attribute. Considering the frequency at which an attribute may change is crucial for determining the most appropriate method.
To sum up, dimensional modelling is an indispensable method for designing data warehouses that ensures rapid and efficient data retrieval. Structuring data into fact and dimension tables not only enhances data accessibility but also supports complex analysis and efficient querying, aiding businesses in deriving actionable insights from their data. Although challenges can arise with data modelling, such as balancing complexity and performance, solutions exist to address these issues. Ultimately, the benefits of implementing dimensional modelling, when it aligns with your business operations, make it a powerful method for any organisation aiming to harness the full potential of its data.
Sources:
https://www.guru99.com/dimensional-model-data-warehouse.html
https://www.thoughtspot.com/data-trends/data-modeling/dimensional-data-modeling
https://blog.devgenius.io/handling-common-challenges-in-dimensional-data-modeling-53ddae86cc06